Research
Hierarchical Latent Class Models for Mortality Surveillance
Overview
Monitoring causes of death is crucial for understanding disease burdens and shaping public health interventions. In many low-resource settings, verbal autopsy (VA) is the primary tool for cause-of-death surveillance, relying on structured interviews with caregivers of the deceased. However, existing VA models often require extensive domain knowledge or large labeled datasets, making them ill-suited for emerging diseases with rapidly evolving epidemiological patterns.
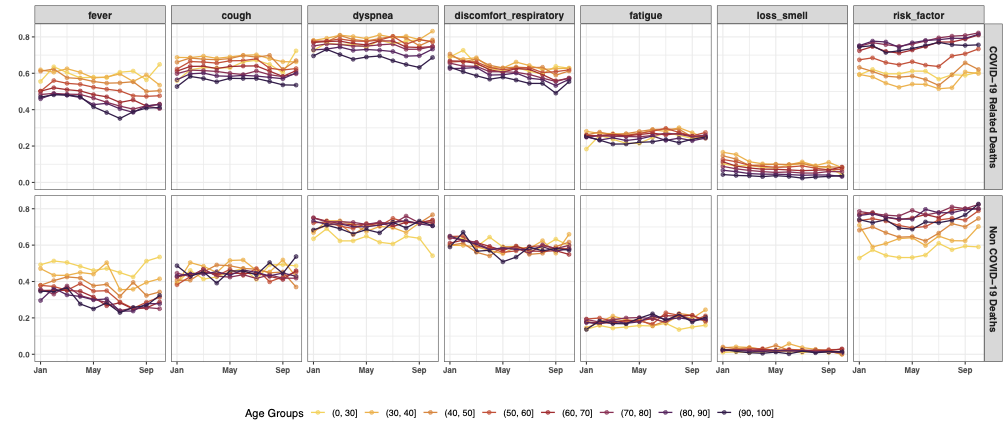
Our Contribution
In our research, we propose a Bayesian hierarchical latent class model that accounts for partially verified VA data, enabling robust cause-of-death estimation under real-world data constraints. Key innovations include:
- Hierarchical Latent Class Modeling
- Captures the joint distribution of symptoms and their shifts over time and across subpopulations.
- Accounts for selective verification bias, a common issue where cause-of-death verification is not randomly assigned.
- Structured Priors for Improved Estimation
- Incorporates domain adaptation techniques to refine estimates for small subpopulations.
- Allows for flexible borrowing of information across related demographic groups.
- Application to COVID-19 Surveillance in Brazil
- We apply our model to COVID-19 mortality data in Brazil (2021), demonstrating how traditional models suffer from bias under selective verification.
- Our method produces more accurate prevalence estimates compared to existing approaches.
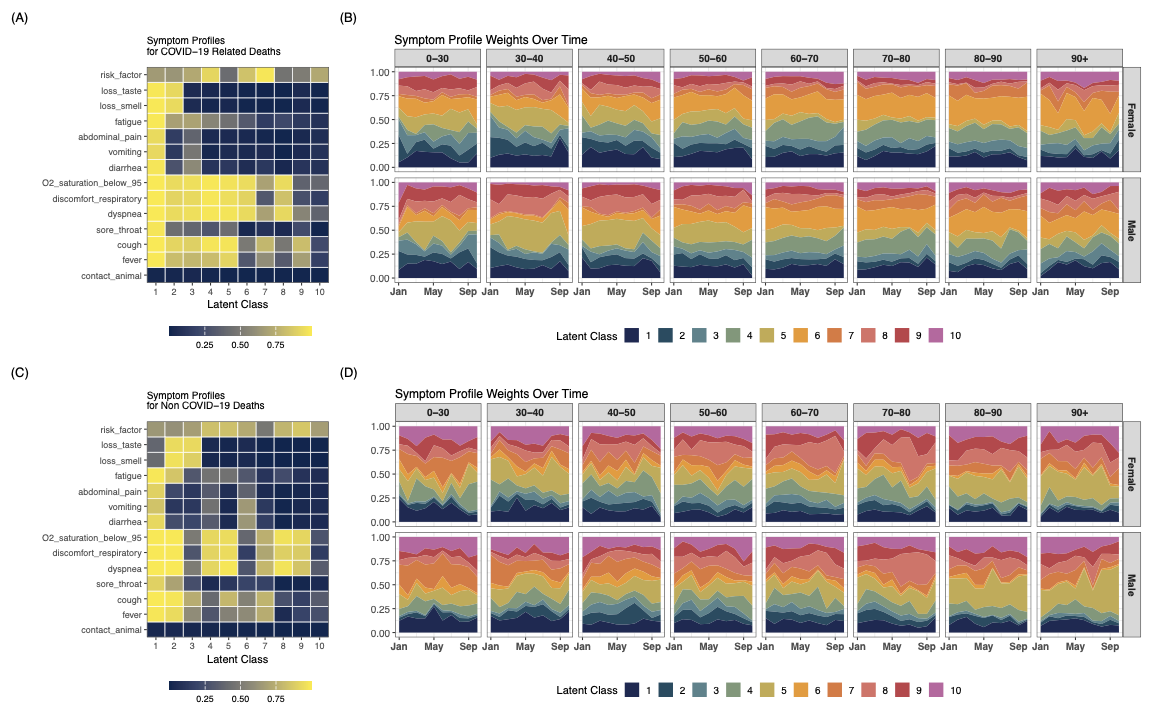
Why This Matters
- Adaptable to Future Public Health Crises
- The framework can be quickly deployed for new diseases where symptom-cause relationships are initially unknown.
- More Reliable Mortality Estimates
- Reduces bias from non-random cause-of-death verification, critical for global disease surveillance.
- Bridging Statistical Innovation with Real-World Impact
- This research integrates Bayesian inference, semi-supervised learning, and domain adaptation to enhance public health decision-making.
Read More
📄 Full Paper: Hierarchical Latent Class Models for Mortality Surveillance (https://arxiv.org/abs/2410.09274)
Treatment Effect Estimation in Dynamic Network Environments [CODE@MIT, 2024]
Overview
Online multiplayer games introduce complex interactions among players, making it difficult to assess the true impact of game features. Traditional A/B testing methods, which assume no interference among units (SUTVA), fail in these settings due to the dynamic and ephemeral network structures formed during gameplay.
In this research, we address the challenges of network interference in online gaming experiments and propose a novel treatment effect estimator that enables post-hoc interference adjustment in randomized experiments.
Key Challenges
- Dynamic Network Structure
- Unlike traditional social networks, online gaming networks continuously change as players match into transient teams.
- Players may participate in multiple games, making their exposure to treatment vary across sessions.
- Violation of SUTVA
- Player experience is influenced by teammates, causing spillover effects that bias traditional A/B testing.
- Treatment assignment may be overwritten by team formation rules, altering true exposure.
- Scalability and Feasibility
- Running cluster-based or hierarchical randomized experiments is computationally expensive and logistically impractical in large-scale online games.
Our Contribution
To address these challenges, we propose a causal inference framework specifically designed for dynamic online gaming environments.
- Novel Treatment Effect Estimation Under Network Interference
- We develop a general exposure mapping function that models how treatment spreads through dynamic team formations.
- Our approach allows post-hoc adjustment of interference while preserving the simplicity of randomized experimental designs.
- Robust Statistical Estimators
- We introduce an estimator that accounts for varying levels of exposure to treatment.
- The method leverages Inverse Probability Weighting (IPW) to correct for biased exposure distributions.
- Empirical Validation with Real Gaming Data
- We test our framework on a Tencent mobile gaming experiment involving 58,565 players over two weeks.
- Results show that naïve estimators significantly underestimate treatment effects, while our method corrects for interference bias.
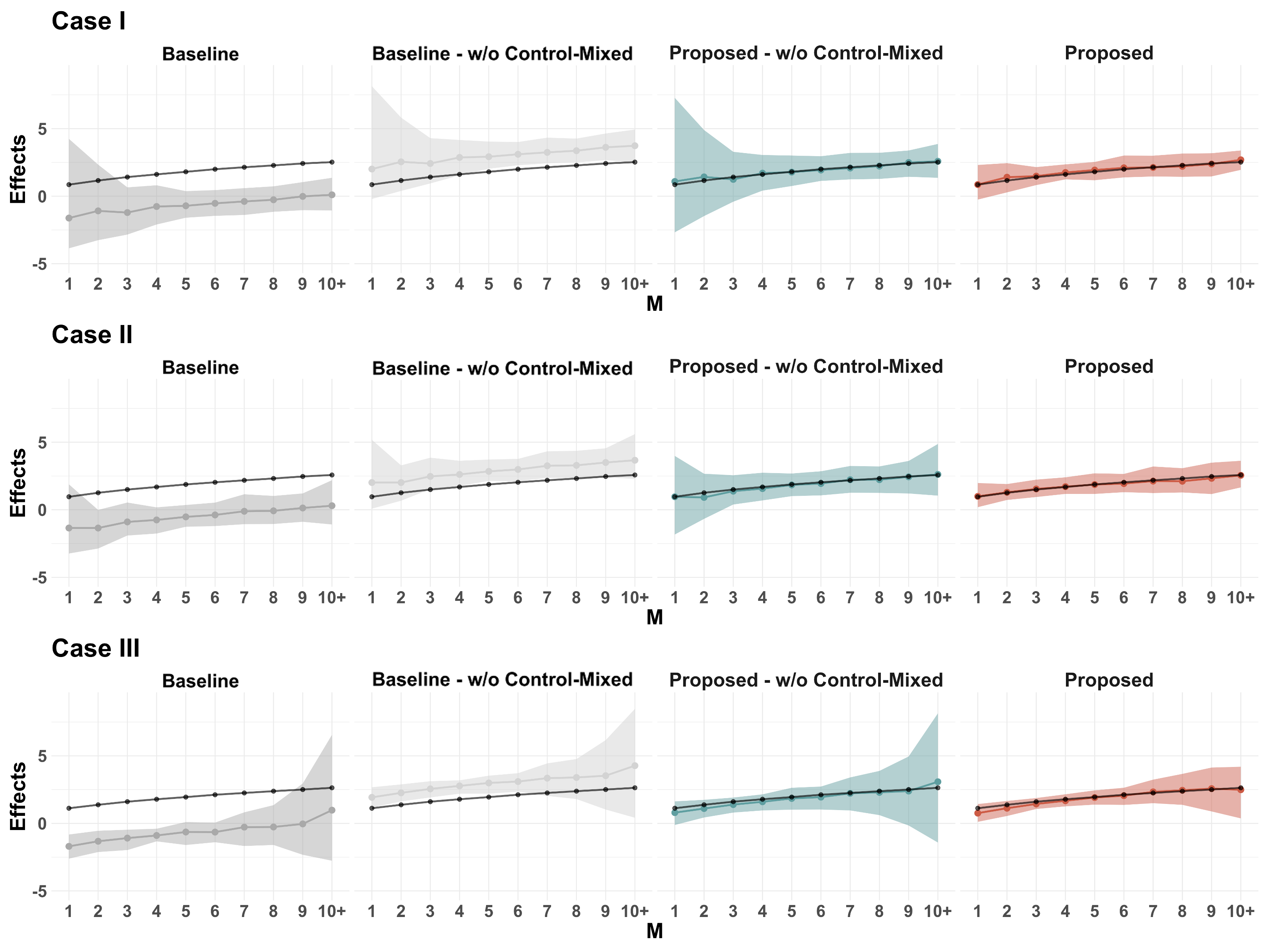
Why This Matters
- Generalizable to Other Dynamic Network Settings
- The framework applies beyond gaming to areas like social media, online marketplaces, and ad campaign experiments.
- Reduces Experimentation Costs
- Enables more efficient experimental designs without needing complex cluster-based interventions.
- Improves Decision-Making in A/B Testing
- More reliable treatment effect estimates help game developers optimize new feature rollouts.
Read More
📄 Full Paper: Treatment Effect Estimation Amidst Dynamic Network Interference (https://arxiv.org/abs/2402.05336)
Bayesian Tensor Decomposition for Verbal Autopsy Analysis
Overview
Accurate cause-of-death estimation is critical for understanding disease burdens and shaping public health policy, especially in low-resource settings where medical certification of deaths is often unavailable. Verbal autopsy (VA) is a widely used alternative, relying on interviews with caregivers to infer the probable cause of death.
Traditional VA models often use latent class models, which assume that symptoms are conditionally independent given the cause of death. However, real-world symptom distributions exhibit complex dependencies, making them hard to interpret and potentially limiting their accuracy.
Our Contribution
We propose a Bayesian hierarchical tensor decomposition framework that balances predictive accuracy with interpretability in VA cause-of-death estimation.
Key innovations include:
- Flexible Probabilistic Tensor Decomposition
- Models grouped symptom dependencies to improve interpretability.
- Uses hierarchical Bayesian modeling for better generalization.
- Dimension-Grouped Factorization
- Introduces r-group independent PARAFACs and c-Tucker decomposition to represent symptom clusters.
- Improves latent class interpretability by structuring sub-profiles of symptoms.
- Scalable Bayesian Inference
- Implements an efficient Markov Chain Monte Carlo (MCMC) approach for model estimation.
- Balances accuracy and computational feasibility for large datasets.
Key Results
We evaluate our method on simulated and real-world VA data, including the PHMRC gold-standard dataset, showing that:
- Our Bayesian tensor decomposition model improves accuracy in cause-of-death estimation.
- Grouping symptoms enhances interpretability without sacrificing predictive power.
- Comparison with baseline models (LCVA, InSilicoVA, standard PARAFAC) confirms our approach is both more robust and generalizable.
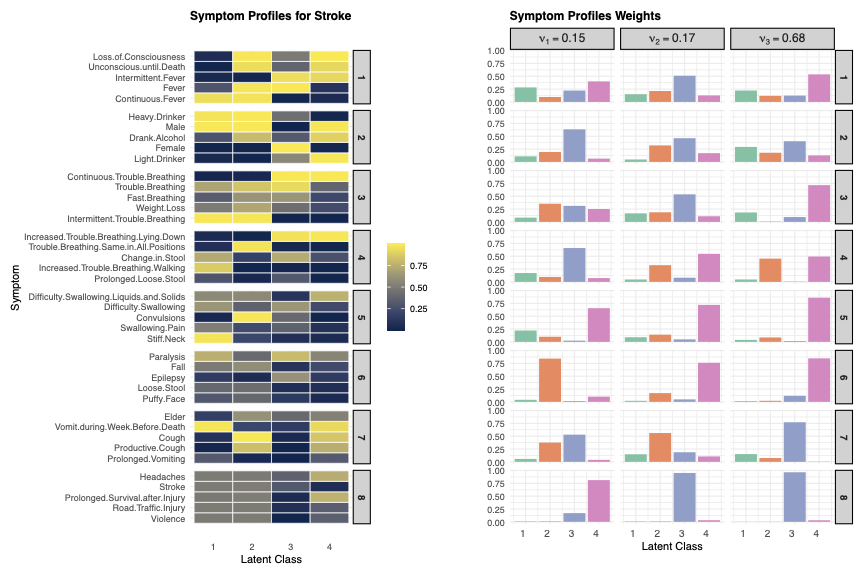
Case Study: PHMRC Gold-Standard VA Dataset
We applied our model to 7,841 adult deaths across 34 causes from the PHMRC dataset, demonstrating:
- Improved cause-of-death classification compared to existing methods.
- Clearer latent symptom clustering, making VA-based mortality modeling more interpretable.
Learned Symptom Clustering
The model identifies distinct symptom groups that correlate with specific diseases, reducing the complexity of high-dimensional VA data.
Cause-of-Death Relationships
Hierarchical clustering of causes based on symptom structures reveals intuitive groupings, such as cardiovascular diseases forming a distinct cluster.
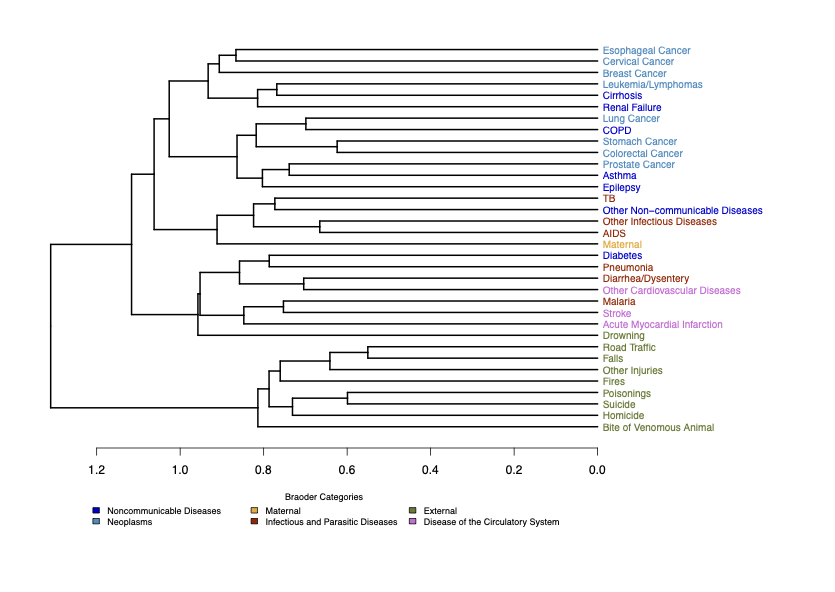
Why This Matters
- More Accurate Mortality Estimates
- Reduces bias from simplistic symptom independence assumptions.
- Improved Interpretability for Public Health Policy
- Helps health organizations better understand disease relationships.
- Scalable Bayesian Framework for Future VA Research
- Enables more generalizable models across different datasets and populations.
Read More
📄 Full Paper: Bayesian Tensor Decomposition for VA (https://arxiv.org/abs/2502.00171)